The security web is abuzz with details about another massive breach.
This time, 27 million data records stored in Suprema, Inc.’s Biostar 2 access control system—including a million peoples’ unencrypted fingerprints and face scan data—were exposed to public access.
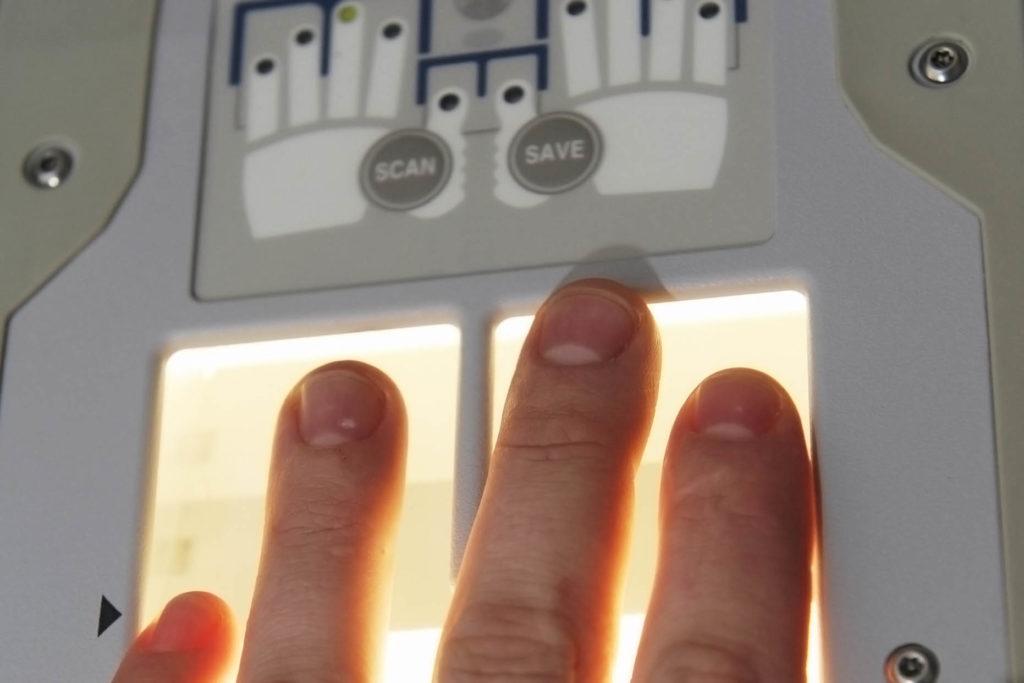
The platform is used to authenticate for and guard access to secure facilities, and was recently integrated with AEOS, which is used by thousands of high-security organizations in dozens of countries.
Traditional Biometrics Puts Privacy and Identity at Risk
The breach demonstrates three key drawbacks of traditional biometric authentication techniques, such as those relying on fingerprints or face scans:
-
Stable data is stored. Fingerprint or face scan data doesn’t change yet must be stored to be used—and as we’ve all seen by now, anything that can be stored can be stolen.
-
Reuse is not impossible. The illicit reuse of fingerprint or face scan data requires only that scanners be fooled by a facsimile for a moment—long enough for access to be granted.
-
The privacy implications are significant. Fingerprints can be traced back to real people and their activities in the real world—and are regularly used for this purpose by much of society.
For these reasons, the storage of fingerprint or face scan data is a risk that many organizations are hesitant to take on—particularly in light of increasingly stringent privacy regulations.
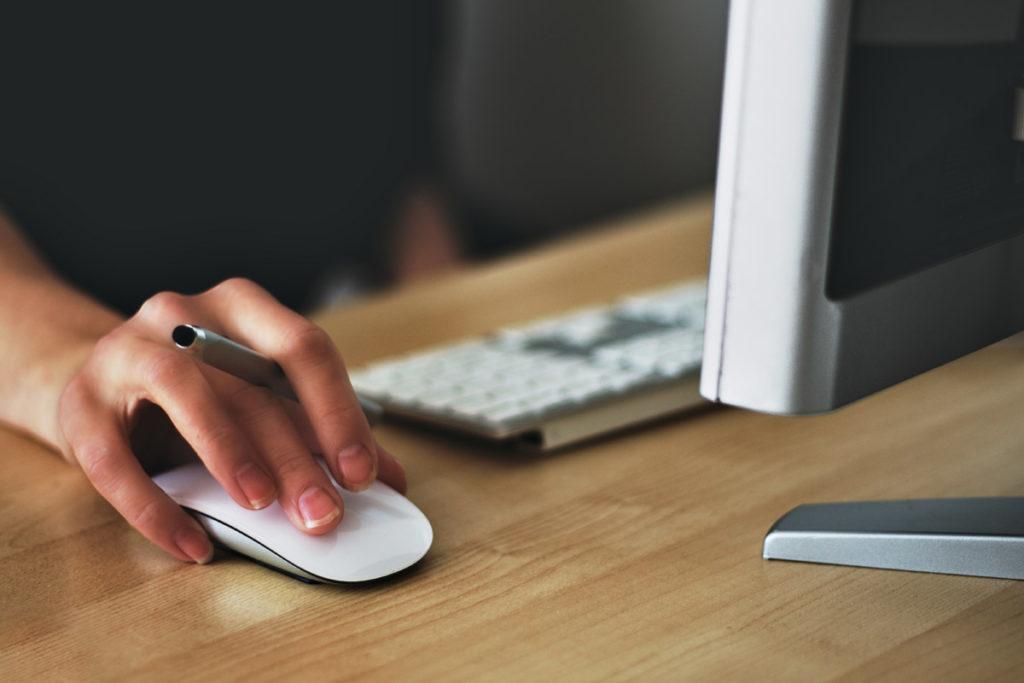
Meanwhile, from the perspective of the individuals affected, a breach like this one is catastrophic—they can’t change their fingerprints or the structures of their faces, yet this data is now out there forever, available for reuse by anyone seeking to impersonate them in an authoritative way.
Behavioral Biometrics is Biometric-Strong, Yet Far Safer
All of this is why behavioral-biometric solutions like Plurilock™ are a far better alternative for multi-factor authentication (MFA) in today’s world.
Traditional biometric tools simply measure the shape of a body part (like a fingerprint) in detail, then authenticate by looking, for a moment, for a matching set of measurements—something that anyone with stolen fingerprint data can plausibly produce, given motivation and resources.
Behavioral biometrics, on the other hand, identifies evolving, yet individual patterns in a user’s bodily movements over time, comparing these to the user’s last known profile of movement patterns. Plurilock’s algorithms, for example, make use of complex micro-patterns in typing and pointer activity for this purpose.
This offers key advantages that shouldn’t be taken lightly:
-
Stored data is not a physical representation. Rather than storing the shape of a fingerprint or face, behavioral biometrics stores numeric timing, position, and statistics data. A stolen fingerprint is relatively easy to re-create. Movement data is different—even if stolen, it is prohibitively difficult to interpret, let alone to replay in order to gain illicit access to protected systems.
-
A match must be sustained for authentication. To reuse a fingerprint, a scanner must only be fooled by a facsimile for a fleeting instant for access to be granted. To fool a behavioral-biometric system, impersonation must be sustained—a far harder task given that it involves deciphering and reusing data about invisible, complex tendencies in bodily movement.
-
Stored data is temporary and evolving. Unlike fingerprints, movement patterns evolve over time as bodies and habits age and change. Behavioral biometrics uses machine learning to enable profiles to evolve in kind, day by day. A somehow-stolen behavioral-biometric profile isn’t of any use months down the road once profile data is out of date and no longer accurate.
-
There are far fewer privacy implications. Data from a somehow-stolen behavioral-biometric profile can’t be traced to any real-world identity, even if deciphered. It’s little more than a bundle of numbers about movements previously made by an anonymous individual, containing no biography or activity details.
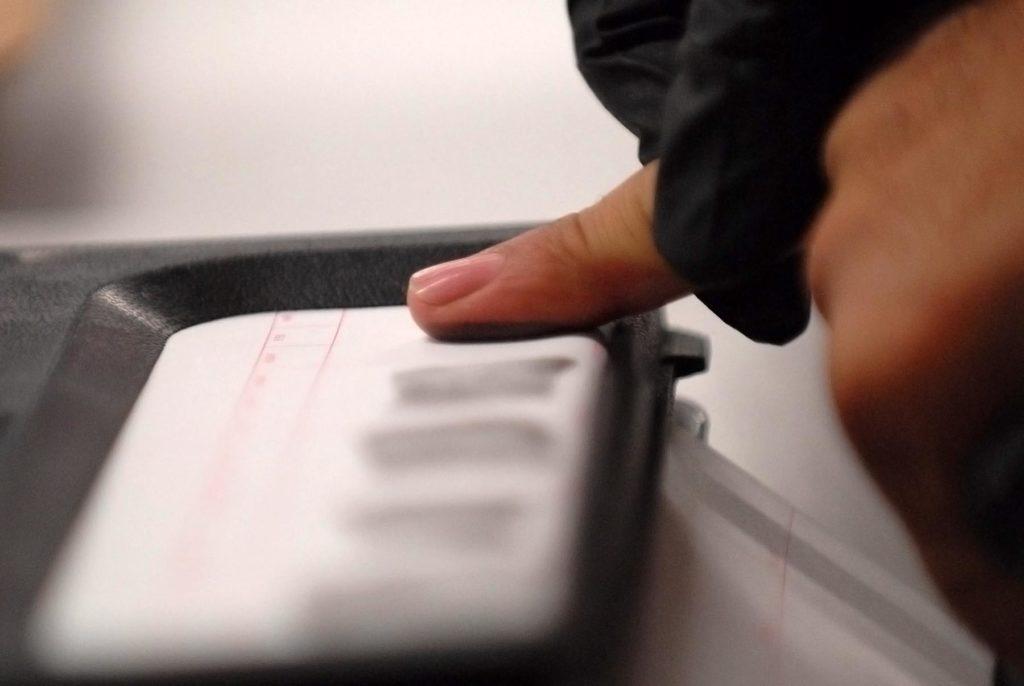
Yes, law enforcement needs permanent, unchanging identity markers to track criminals responsible for crime scenes and so on—but the same properties that make traditional biometrics great for police work make these tools a privacy nightmare for everyday authentication in computing.
Behavioral biometrics is a far better solution for the latter purpose, and is also a stronger authenticator than fingerprint and face scans, both of which are considerably easier to impersonate.
Machine Learning Means More Signals
Even better, since machine learning techniques are intrinsic to behavioral-biometric systems, they can be used to consider patterns in other signals for authentication decisions as well.
In Plurilock’s case, we augment analysis of behavioral-biometric movement data with additional identifying patterns in:
-
Location and travel
-
Network context
-
Endpoint characteristics and fingerprinting
-
Other observable data
Such data can be analyzed by Plurilock’s machine learning engine in much the same way that bodily movement data can, and with many of the same privacy benefits—not storage of actual activity or identity data, but rather of the intricate statistics that characterize them over time.
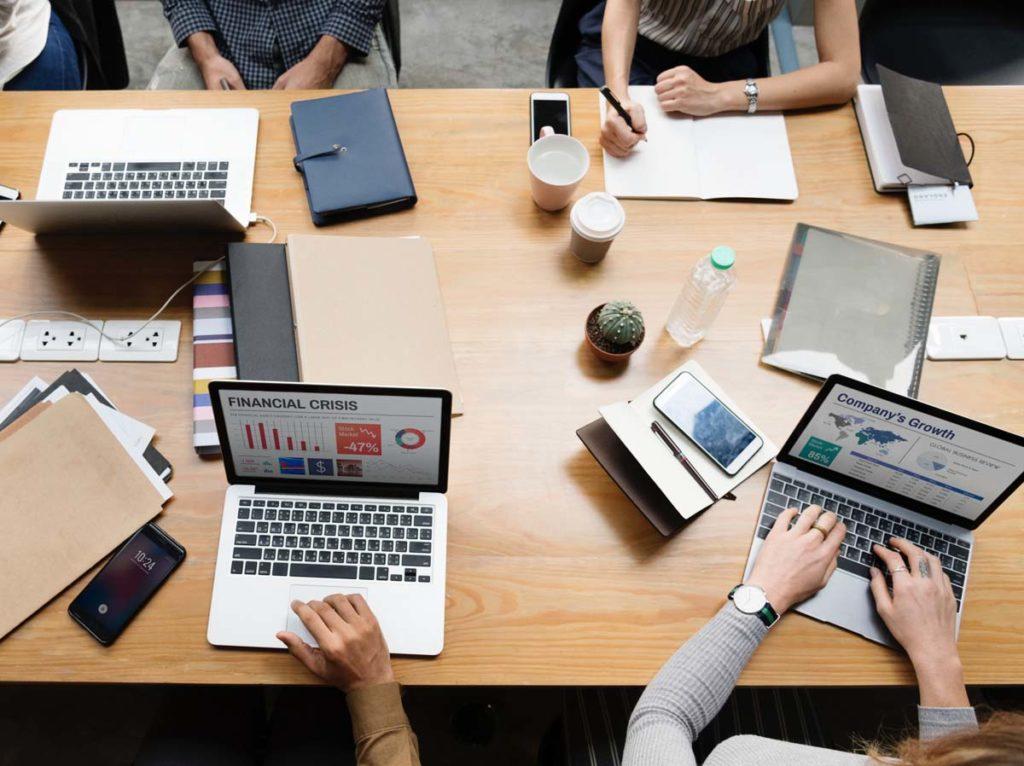
By augmenting movement biometrics with additional signals, the result is a very strong, biometric form of authentication that’s impossible to fool, yet presents few if any privacy risks in the unlikely event that anonymous profile data is somehow stolen and decrypted.
Biometrics Yes, Fingerprints No
We think that the impulse toward biometric multi-factor authentication (MFA) isn’t just a good one, but an essential one, given the rates of data breach and theft that we see today.
The case of the Biostar 2 data breach, however, shows why using traditional fingerprint or face-scan biometrics are a risky proposition. Millions of users may face highly vexing identity issues for years to come as a result of the incident.
Today’s networked, sensor-laden, machine-learning world is a perfect match for far stronger, safer, and more secure behavioral-biometric technologies that analyze dynamic, evolving patterns in motion rather than merely measuring and storing identifying details about parts of bodies.
Our bias is showing, but here at Plurilock, we strongly encourage you to find and deploy a behavioral-biometric technology as your MFA solution. ■